Would this neural network have short term memory?Arbitrarily big neural networkWhy would neural networks be a...
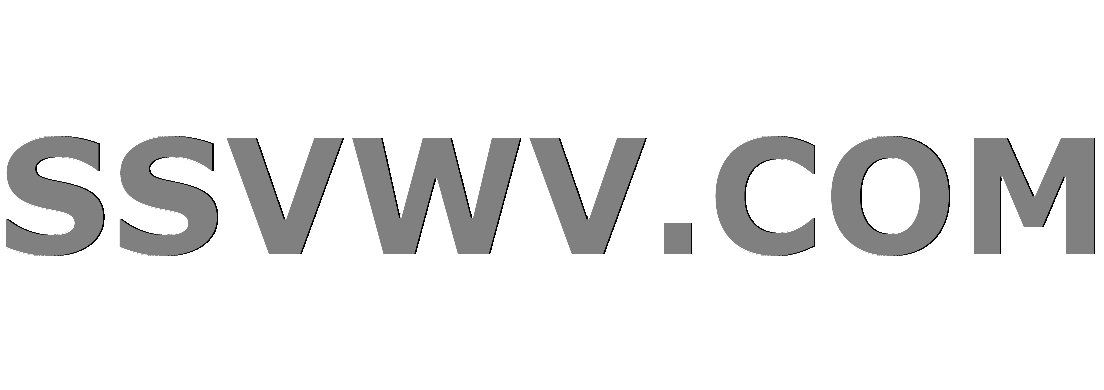
Multi tool use
Can I make a matrix from just a parts of the cells?
Does academia have a lazy work culture?
Why doesn't current cancel in a generator?
Why are so many countries still in the Commonwealth?
How do we explain the E major chord in this progression?
Is there a reason why I should not use the HaveIBeenPwned API to warn users about exposed passwords?
How to deal with a player who makes bad characters and kills them?
How can I prevent corporations from growing their own workforce?
Why is drive/partition number still used?
Keeping an "hot eyeball planet" wet
How do I run a game when my PCs have different approaches to combat?
Iterate over non-const variables in C++
Decreasing star size
AC contactor 1 pole or 2?
Spoken encryption
Examples of simultaneous independent breakthroughs
Why/when is AC-DC-AC conversion superior to direct AC-Ac conversion?
Does the Intel 8086 CPU have user mode and kernel mode?
Basic Questions on Wiener Filtering
Why was Sauron preparing for war instead of trying to find the ring?
Problem in styling a monochrome plot
How do I stop my characters falling in love?
Can two figures have the same area, perimeter, and same number of segments have different shape?
What's the difference between 2a and 10a charging options?
Would this neural network have short term memory?
Arbitrarily big neural networkWhy would neural networks be a particularly good framework for “embodied AI”?If a neural network approach becomes widely used within a real-world situation, how would one debug/understand/fix the outcome if in one case poor?Detect street and sidewalk surface in aerial imagery (neural network)When do you back-propagate errors through a Neural Network when using TD LambdaHow to create a task-graph based neural network?Why not teach to a NN not only what is true, but also what is not true?Neural Network for Optical Mark Recognition?Using an 'operation ID' as a neural network inputWould this NN for my chip outputs work?
.everyoneloves__top-leaderboard:empty,.everyoneloves__mid-leaderboard:empty,.everyoneloves__bot-mid-leaderboard:empty{ margin-bottom:0;
}
$begingroup$
I want to design a NN that can remember it's last 7 actions and use them as inputs. So for example it would be able to store words in it's memory. Therefore if it had a choice of 10 different actions, the number of words it could store is $10^7$.
Here is my design:
$$out_{n+1} = f(out_n, in_n)mathbf{N} + out_n.mathbf{M}$$
$$action_n = sigma(mathbf{N} cdot out_n)$$
Where $f$ represents some layered neural network. Some of the actions would be physical actions and some might be internal (such as thinking of the letter 'C').
Basically I want $out_n$ to be an array that keeps the last 6 action values and puts them back in. So $M$ will be the matrix:
$$begin{bmatrix}
0&1&0&0&0&0\
0&0&1&0&0&0\
0&0&0&1&0&0\
0&0&0&0&1&0\
0&0&0&0&0&1\
0&0&0&0&0&0
end{bmatrix}$$
i.e. it would drop the 6th item from it's memory.
and $N$ would be the vector:
$$begin{bmatrix}
1&0&0&0&0&0&0
end{bmatrix}$$
I think this would be equivalent to an equation of the form:
$$out_{n+1}=F(in_n,out_n,out_{n-1},out_{n-2},...,out_{n-6})$$
So I think this would be an advantage over an RNN since this model remembers precisely it's last 6 actions. But would this be better than an RNN or worse? One could increase it's memory to more than 7 quite easily.
I think it's basically the same archececture as an RNN except elinimating a lot of the connections. Is this a new design or a common design?
One problem with this design is that you might also want a memory that is over longer time periods (e.g. for actions that take more than one tick.) But that might be solved by enhancing the archecture.
neural-networks long-short-term-memory
$endgroup$
add a comment |
$begingroup$
I want to design a NN that can remember it's last 7 actions and use them as inputs. So for example it would be able to store words in it's memory. Therefore if it had a choice of 10 different actions, the number of words it could store is $10^7$.
Here is my design:
$$out_{n+1} = f(out_n, in_n)mathbf{N} + out_n.mathbf{M}$$
$$action_n = sigma(mathbf{N} cdot out_n)$$
Where $f$ represents some layered neural network. Some of the actions would be physical actions and some might be internal (such as thinking of the letter 'C').
Basically I want $out_n$ to be an array that keeps the last 6 action values and puts them back in. So $M$ will be the matrix:
$$begin{bmatrix}
0&1&0&0&0&0\
0&0&1&0&0&0\
0&0&0&1&0&0\
0&0&0&0&1&0\
0&0&0&0&0&1\
0&0&0&0&0&0
end{bmatrix}$$
i.e. it would drop the 6th item from it's memory.
and $N$ would be the vector:
$$begin{bmatrix}
1&0&0&0&0&0&0
end{bmatrix}$$
I think this would be equivalent to an equation of the form:
$$out_{n+1}=F(in_n,out_n,out_{n-1},out_{n-2},...,out_{n-6})$$
So I think this would be an advantage over an RNN since this model remembers precisely it's last 6 actions. But would this be better than an RNN or worse? One could increase it's memory to more than 7 quite easily.
I think it's basically the same archececture as an RNN except elinimating a lot of the connections. Is this a new design or a common design?
One problem with this design is that you might also want a memory that is over longer time periods (e.g. for actions that take more than one tick.) But that might be solved by enhancing the archecture.
neural-networks long-short-term-memory
$endgroup$
add a comment |
$begingroup$
I want to design a NN that can remember it's last 7 actions and use them as inputs. So for example it would be able to store words in it's memory. Therefore if it had a choice of 10 different actions, the number of words it could store is $10^7$.
Here is my design:
$$out_{n+1} = f(out_n, in_n)mathbf{N} + out_n.mathbf{M}$$
$$action_n = sigma(mathbf{N} cdot out_n)$$
Where $f$ represents some layered neural network. Some of the actions would be physical actions and some might be internal (such as thinking of the letter 'C').
Basically I want $out_n$ to be an array that keeps the last 6 action values and puts them back in. So $M$ will be the matrix:
$$begin{bmatrix}
0&1&0&0&0&0\
0&0&1&0&0&0\
0&0&0&1&0&0\
0&0&0&0&1&0\
0&0&0&0&0&1\
0&0&0&0&0&0
end{bmatrix}$$
i.e. it would drop the 6th item from it's memory.
and $N$ would be the vector:
$$begin{bmatrix}
1&0&0&0&0&0&0
end{bmatrix}$$
I think this would be equivalent to an equation of the form:
$$out_{n+1}=F(in_n,out_n,out_{n-1},out_{n-2},...,out_{n-6})$$
So I think this would be an advantage over an RNN since this model remembers precisely it's last 6 actions. But would this be better than an RNN or worse? One could increase it's memory to more than 7 quite easily.
I think it's basically the same archececture as an RNN except elinimating a lot of the connections. Is this a new design or a common design?
One problem with this design is that you might also want a memory that is over longer time periods (e.g. for actions that take more than one tick.) But that might be solved by enhancing the archecture.
neural-networks long-short-term-memory
$endgroup$
I want to design a NN that can remember it's last 7 actions and use them as inputs. So for example it would be able to store words in it's memory. Therefore if it had a choice of 10 different actions, the number of words it could store is $10^7$.
Here is my design:
$$out_{n+1} = f(out_n, in_n)mathbf{N} + out_n.mathbf{M}$$
$$action_n = sigma(mathbf{N} cdot out_n)$$
Where $f$ represents some layered neural network. Some of the actions would be physical actions and some might be internal (such as thinking of the letter 'C').
Basically I want $out_n$ to be an array that keeps the last 6 action values and puts them back in. So $M$ will be the matrix:
$$begin{bmatrix}
0&1&0&0&0&0\
0&0&1&0&0&0\
0&0&0&1&0&0\
0&0&0&0&1&0\
0&0&0&0&0&1\
0&0&0&0&0&0
end{bmatrix}$$
i.e. it would drop the 6th item from it's memory.
and $N$ would be the vector:
$$begin{bmatrix}
1&0&0&0&0&0&0
end{bmatrix}$$
I think this would be equivalent to an equation of the form:
$$out_{n+1}=F(in_n,out_n,out_{n-1},out_{n-2},...,out_{n-6})$$
So I think this would be an advantage over an RNN since this model remembers precisely it's last 6 actions. But would this be better than an RNN or worse? One could increase it's memory to more than 7 quite easily.
I think it's basically the same archececture as an RNN except elinimating a lot of the connections. Is this a new design or a common design?
One problem with this design is that you might also want a memory that is over longer time periods (e.g. for actions that take more than one tick.) But that might be solved by enhancing the archecture.
neural-networks long-short-term-memory
neural-networks long-short-term-memory
edited 7 hours ago
zooby
asked 8 hours ago


zoobyzooby
6564 silver badges12 bronze badges
6564 silver badges12 bronze badges
add a comment |
add a comment |
1 Answer
1
active
oldest
votes
$begingroup$
Congrats, you have invented 1d convolution. Convolution combined with RNN would have some advantage over just RNN. Think about the perception field.
In this layer, you do aggregate $6$ values to one. Imagine two of them - it will be $36$ already, etc. But, in the end, you still need RNN at the end to aggregate a variable length to constant length.
$endgroup$
$begingroup$
Well that's good! Glad I'm on the right track! (Not sure what you mean at the end about variable lengths).
$endgroup$
– zooby
7 hours ago
$begingroup$
@zooby This is not a 1D CNN, its a non differentiable RNN. (actions must be sampled under some categorical distribution based on whats described). The only similarity to a 1d cnn is the sliding window
$endgroup$
– mshlis
7 hours ago
$begingroup$
Why is it non-differentiable ?
$endgroup$
– zooby
7 hours ago
$begingroup$
do you train with sequences of different lenght, right? also if you put output as input think about output may be wrong so you can consider to force-feeding ( expected data instead of output)
$endgroup$
– user8426627
6 hours ago
$begingroup$
I could be wrong but generally actions are drawn from a distribution (that’s why you show one hot encodingns) and you can’t differentiate through a categorical distrib
$endgroup$
– mshlis
6 hours ago
add a comment |
Your Answer
StackExchange.ready(function() {
var channelOptions = {
tags: "".split(" "),
id: "658"
};
initTagRenderer("".split(" "), "".split(" "), channelOptions);
StackExchange.using("externalEditor", function() {
// Have to fire editor after snippets, if snippets enabled
if (StackExchange.settings.snippets.snippetsEnabled) {
StackExchange.using("snippets", function() {
createEditor();
});
}
else {
createEditor();
}
});
function createEditor() {
StackExchange.prepareEditor({
heartbeatType: 'answer',
autoActivateHeartbeat: false,
convertImagesToLinks: false,
noModals: true,
showLowRepImageUploadWarning: true,
reputationToPostImages: null,
bindNavPrevention: true,
postfix: "",
imageUploader: {
brandingHtml: "Powered by u003ca class="icon-imgur-white" href="https://imgur.com/"u003eu003c/au003e",
contentPolicyHtml: "User contributions licensed under u003ca href="https://creativecommons.org/licenses/by-sa/3.0/"u003ecc by-sa 3.0 with attribution requiredu003c/au003e u003ca href="https://stackoverflow.com/legal/content-policy"u003e(content policy)u003c/au003e",
allowUrls: true
},
noCode: true, onDemand: true,
discardSelector: ".discard-answer"
,immediatelyShowMarkdownHelp:true
});
}
});
Sign up or log in
StackExchange.ready(function () {
StackExchange.helpers.onClickDraftSave('#login-link');
});
Sign up using Google
Sign up using Facebook
Sign up using Email and Password
Post as a guest
Required, but never shown
StackExchange.ready(
function () {
StackExchange.openid.initPostLogin('.new-post-login', 'https%3a%2f%2fai.stackexchange.com%2fquestions%2f13622%2fwould-this-neural-network-have-short-term-memory%23new-answer', 'question_page');
}
);
Post as a guest
Required, but never shown
1 Answer
1
active
oldest
votes
1 Answer
1
active
oldest
votes
active
oldest
votes
active
oldest
votes
$begingroup$
Congrats, you have invented 1d convolution. Convolution combined with RNN would have some advantage over just RNN. Think about the perception field.
In this layer, you do aggregate $6$ values to one. Imagine two of them - it will be $36$ already, etc. But, in the end, you still need RNN at the end to aggregate a variable length to constant length.
$endgroup$
$begingroup$
Well that's good! Glad I'm on the right track! (Not sure what you mean at the end about variable lengths).
$endgroup$
– zooby
7 hours ago
$begingroup$
@zooby This is not a 1D CNN, its a non differentiable RNN. (actions must be sampled under some categorical distribution based on whats described). The only similarity to a 1d cnn is the sliding window
$endgroup$
– mshlis
7 hours ago
$begingroup$
Why is it non-differentiable ?
$endgroup$
– zooby
7 hours ago
$begingroup$
do you train with sequences of different lenght, right? also if you put output as input think about output may be wrong so you can consider to force-feeding ( expected data instead of output)
$endgroup$
– user8426627
6 hours ago
$begingroup$
I could be wrong but generally actions are drawn from a distribution (that’s why you show one hot encodingns) and you can’t differentiate through a categorical distrib
$endgroup$
– mshlis
6 hours ago
add a comment |
$begingroup$
Congrats, you have invented 1d convolution. Convolution combined with RNN would have some advantage over just RNN. Think about the perception field.
In this layer, you do aggregate $6$ values to one. Imagine two of them - it will be $36$ already, etc. But, in the end, you still need RNN at the end to aggregate a variable length to constant length.
$endgroup$
$begingroup$
Well that's good! Glad I'm on the right track! (Not sure what you mean at the end about variable lengths).
$endgroup$
– zooby
7 hours ago
$begingroup$
@zooby This is not a 1D CNN, its a non differentiable RNN. (actions must be sampled under some categorical distribution based on whats described). The only similarity to a 1d cnn is the sliding window
$endgroup$
– mshlis
7 hours ago
$begingroup$
Why is it non-differentiable ?
$endgroup$
– zooby
7 hours ago
$begingroup$
do you train with sequences of different lenght, right? also if you put output as input think about output may be wrong so you can consider to force-feeding ( expected data instead of output)
$endgroup$
– user8426627
6 hours ago
$begingroup$
I could be wrong but generally actions are drawn from a distribution (that’s why you show one hot encodingns) and you can’t differentiate through a categorical distrib
$endgroup$
– mshlis
6 hours ago
add a comment |
$begingroup$
Congrats, you have invented 1d convolution. Convolution combined with RNN would have some advantage over just RNN. Think about the perception field.
In this layer, you do aggregate $6$ values to one. Imagine two of them - it will be $36$ already, etc. But, in the end, you still need RNN at the end to aggregate a variable length to constant length.
$endgroup$
Congrats, you have invented 1d convolution. Convolution combined with RNN would have some advantage over just RNN. Think about the perception field.
In this layer, you do aggregate $6$ values to one. Imagine two of them - it will be $36$ already, etc. But, in the end, you still need RNN at the end to aggregate a variable length to constant length.
edited 4 hours ago


nbro
5,6604 gold badges15 silver badges32 bronze badges
5,6604 gold badges15 silver badges32 bronze badges
answered 8 hours ago
user8426627user8426627
22411 bronze badges
22411 bronze badges
$begingroup$
Well that's good! Glad I'm on the right track! (Not sure what you mean at the end about variable lengths).
$endgroup$
– zooby
7 hours ago
$begingroup$
@zooby This is not a 1D CNN, its a non differentiable RNN. (actions must be sampled under some categorical distribution based on whats described). The only similarity to a 1d cnn is the sliding window
$endgroup$
– mshlis
7 hours ago
$begingroup$
Why is it non-differentiable ?
$endgroup$
– zooby
7 hours ago
$begingroup$
do you train with sequences of different lenght, right? also if you put output as input think about output may be wrong so you can consider to force-feeding ( expected data instead of output)
$endgroup$
– user8426627
6 hours ago
$begingroup$
I could be wrong but generally actions are drawn from a distribution (that’s why you show one hot encodingns) and you can’t differentiate through a categorical distrib
$endgroup$
– mshlis
6 hours ago
add a comment |
$begingroup$
Well that's good! Glad I'm on the right track! (Not sure what you mean at the end about variable lengths).
$endgroup$
– zooby
7 hours ago
$begingroup$
@zooby This is not a 1D CNN, its a non differentiable RNN. (actions must be sampled under some categorical distribution based on whats described). The only similarity to a 1d cnn is the sliding window
$endgroup$
– mshlis
7 hours ago
$begingroup$
Why is it non-differentiable ?
$endgroup$
– zooby
7 hours ago
$begingroup$
do you train with sequences of different lenght, right? also if you put output as input think about output may be wrong so you can consider to force-feeding ( expected data instead of output)
$endgroup$
– user8426627
6 hours ago
$begingroup$
I could be wrong but generally actions are drawn from a distribution (that’s why you show one hot encodingns) and you can’t differentiate through a categorical distrib
$endgroup$
– mshlis
6 hours ago
$begingroup$
Well that's good! Glad I'm on the right track! (Not sure what you mean at the end about variable lengths).
$endgroup$
– zooby
7 hours ago
$begingroup$
Well that's good! Glad I'm on the right track! (Not sure what you mean at the end about variable lengths).
$endgroup$
– zooby
7 hours ago
$begingroup$
@zooby This is not a 1D CNN, its a non differentiable RNN. (actions must be sampled under some categorical distribution based on whats described). The only similarity to a 1d cnn is the sliding window
$endgroup$
– mshlis
7 hours ago
$begingroup$
@zooby This is not a 1D CNN, its a non differentiable RNN. (actions must be sampled under some categorical distribution based on whats described). The only similarity to a 1d cnn is the sliding window
$endgroup$
– mshlis
7 hours ago
$begingroup$
Why is it non-differentiable ?
$endgroup$
– zooby
7 hours ago
$begingroup$
Why is it non-differentiable ?
$endgroup$
– zooby
7 hours ago
$begingroup$
do you train with sequences of different lenght, right? also if you put output as input think about output may be wrong so you can consider to force-feeding ( expected data instead of output)
$endgroup$
– user8426627
6 hours ago
$begingroup$
do you train with sequences of different lenght, right? also if you put output as input think about output may be wrong so you can consider to force-feeding ( expected data instead of output)
$endgroup$
– user8426627
6 hours ago
$begingroup$
I could be wrong but generally actions are drawn from a distribution (that’s why you show one hot encodingns) and you can’t differentiate through a categorical distrib
$endgroup$
– mshlis
6 hours ago
$begingroup$
I could be wrong but generally actions are drawn from a distribution (that’s why you show one hot encodingns) and you can’t differentiate through a categorical distrib
$endgroup$
– mshlis
6 hours ago
add a comment |
Thanks for contributing an answer to Artificial Intelligence Stack Exchange!
- Please be sure to answer the question. Provide details and share your research!
But avoid …
- Asking for help, clarification, or responding to other answers.
- Making statements based on opinion; back them up with references or personal experience.
Use MathJax to format equations. MathJax reference.
To learn more, see our tips on writing great answers.
Sign up or log in
StackExchange.ready(function () {
StackExchange.helpers.onClickDraftSave('#login-link');
});
Sign up using Google
Sign up using Facebook
Sign up using Email and Password
Post as a guest
Required, but never shown
StackExchange.ready(
function () {
StackExchange.openid.initPostLogin('.new-post-login', 'https%3a%2f%2fai.stackexchange.com%2fquestions%2f13622%2fwould-this-neural-network-have-short-term-memory%23new-answer', 'question_page');
}
);
Post as a guest
Required, but never shown
Sign up or log in
StackExchange.ready(function () {
StackExchange.helpers.onClickDraftSave('#login-link');
});
Sign up using Google
Sign up using Facebook
Sign up using Email and Password
Post as a guest
Required, but never shown
Sign up or log in
StackExchange.ready(function () {
StackExchange.helpers.onClickDraftSave('#login-link');
});
Sign up using Google
Sign up using Facebook
Sign up using Email and Password
Post as a guest
Required, but never shown
Sign up or log in
StackExchange.ready(function () {
StackExchange.helpers.onClickDraftSave('#login-link');
});
Sign up using Google
Sign up using Facebook
Sign up using Email and Password
Sign up using Google
Sign up using Facebook
Sign up using Email and Password
Post as a guest
Required, but never shown
Required, but never shown
Required, but never shown
Required, but never shown
Required, but never shown
Required, but never shown
Required, but never shown
Required, but never shown
Required, but never shown
xnfb,wA8QJUpEltYVAYo41nguiRJK9ENmR7,lI